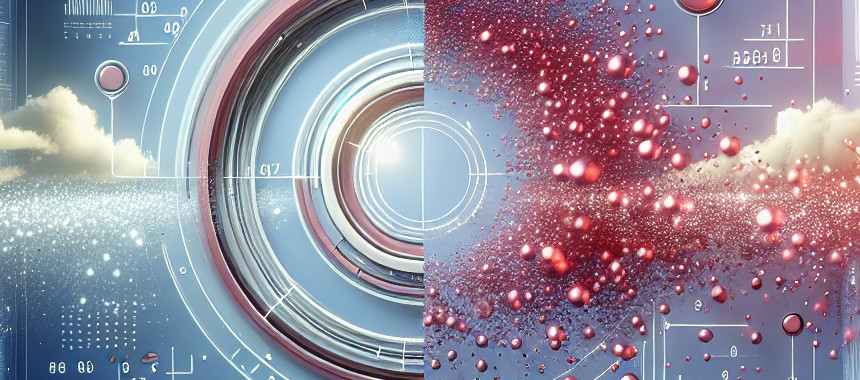
Data Annotation Report
: Analysis on the Market, Trends, and TechnologiesThe data annotation sector is a critical component in the advancement of artificial intelligence (AI), machine learning (ML), and big data analytics. The rise of data annotation is closely tied to the development of AI and ML models, which require vast amounts of accurately labeled data to train algorithms. As a result, companies are seeking high-quality data annotation services to enhance decision-making and foster technological innovation. The sector’s expansion is characterized by a focus on various data types, including image, video, audio, and text annotation, and is driven by the need for precision, scalability, and cost-effectiveness. This data annotation report explores the growing ecosystem of data annotation where companies offer a multitude of applications, from autonomous vehicles to healthcare analytics. This demonstrates the sector’s critical role in shaping the future of AI and data management.
We updated this report 110 days ago. Noticed something’s off? Let’s make it right together — reach out!
Topic Dominance Index of Data Annotation
To identify the Dominance Index of Data Annotation in the Trend and Technology ecosystem, we look at 3 different time series: the timeline of published articles, founded companies, and global search. These timelines are normalized and combined to show a comprehensive view of the Data Annotation evolution relative to all known Trends and Technologies.
Key Activities and Applications
- Autonomous Vehicles: Data annotation for autonomous driving systems is gaining momentum, including image and video labeling for object recognition.
- Quality Control & Validation: Ensuring the accuracy of annotations using automated tools and human judgment to identify and correct errors or inconsistencies.
- Semantic Annotation: Identifying relationships between annotated elements or providing contextual descriptions that allow AI models to better understand labels.
- Text Annotation for NLP: Annotating text data for tasks like sentiment analysis, entity recognition, or machine translation involves tagging parts of text according to their linguistic or semantic properties.
- Crowdsourcing & Collaborative Annotation: Managing large-scale annotation projects by distributing the work among multiple annotators through crowdsourcing platforms, while maintaining dataset consistency and quality.
- Data Enrichment: Augmenting data with metadata, synthesizing data to increase variability, or merging datasets for a broader scope.
Emergent Trends and Core Insights
- Unbiased Datasets: Increased demand for diverse and unbiased datasets to avoid AI algorithmic biases.
- Synthetic Data: Growth in the use of synthetic data to supplement real-world data annotation efforts to increase variability.
- Automation with Human-in-the-Loop: Automating the annotation process using AI tools, with human annotators involved for quality control and handling complex cases.
- Privacy-Preserving Annotation: Techniques like differential privacy and secure multi-party computation are being explored to annotate data without exposing sensitive information.
- Domain-Specific Annotation: Tailored data annotation solutions for specific industries and applications, such as healthcare, autonomous vehicles, or finance.
- Multimodal Data Annotation: With AI models increasingly handling multiple types of data (e.g., combining text, images, and audio), there is a trend towards synchronizing annotations across different data types to provide richer training data.
Technologies and Methodologies
- AI-Assisted Annotation: Leveraging AI and machine learning models to pre-annotate data based on previous patterns and examples. These systems learn from past annotations and provide accurate suggestions.
- Cloud Computing: Implementation of data annotation solutions in the cloud ensures secure and collaborative annotation workflows.
- Computer Vision: Integration of advanced computer vision technologies for precise image and video annotation.
- Natural Language Processing (NLP): Automatically identifies and tags parts of speech, sentiment, named entities, and other linguistic features to accelerate the annotation process for large text datasets.
- Quality Control Algorithms: Monitor the consistency and accuracy of annotations across different annotators using techniques like inter-annotator agreement metrics.
- Transfer Learning & Pretrained Models: Provide a head start in the annotation process, especially for complex tasks like image segmentation or entity recognition.
Data Annotation Funding
A total of 118 Data Annotation companies have received funding.
Overall, Data Annotation companies have raised $1.5B.
Companies within the Data Annotation domain have secured capital from 283 funding rounds.
The chart shows the funding trendline of Data Annotation companies over the last 5 years
Data Annotation Companies
The Companies feature is a crucial part of TrendFeedr. It offers in-depth information about 571 companies working within Data Annotation and other trends and technologies. Identify and analyze innovators and key players in relevant industries more easily with this feature.
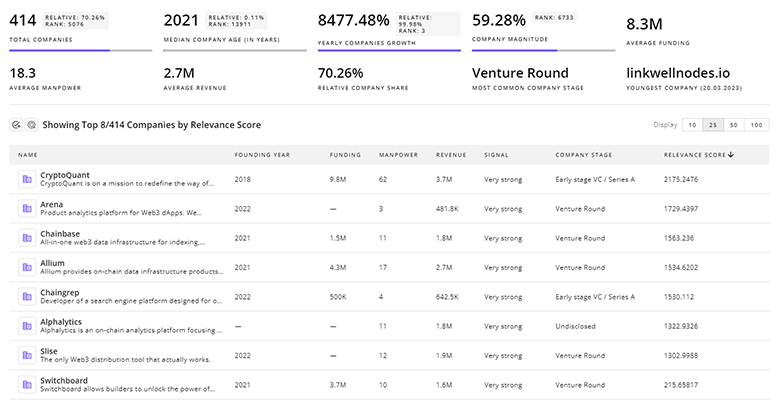
571 Data Annotation Companies
Discover Data Annotation Companies, their Funding, Manpower, Revenues, Stages, and much more
Data Annotation Investors
TrendFeedr’s investors tool offers a detailed view of investment activities that align with specific trends and technologies. This tool features comprehensive data on 126 Data Annotation investors, funding rounds, and investment trends, providing an overview of market dynamics.
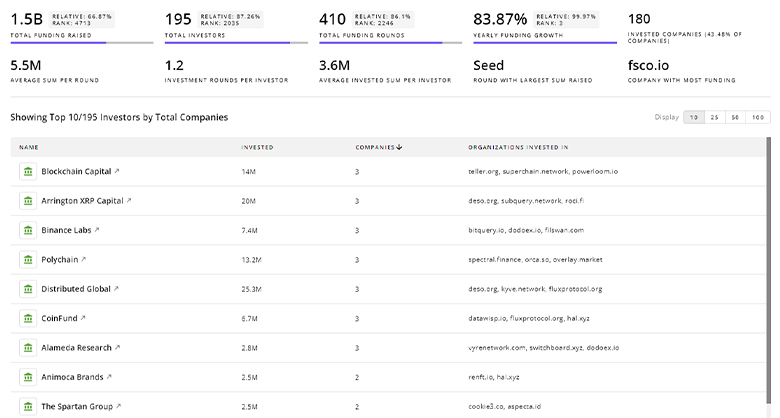
126 Data Annotation Investors
Discover Data Annotation Investors, Funding Rounds, Invested Amounts, and Funding Growth
Data Annotation News
Stay informed and ahead of the curve with TrendFeedr’s News feature, which provides access to 1.7K Data Annotation articles. The tool is tailored for professionals seeking to understand the historical trajectory and current momentum of changing market trends.
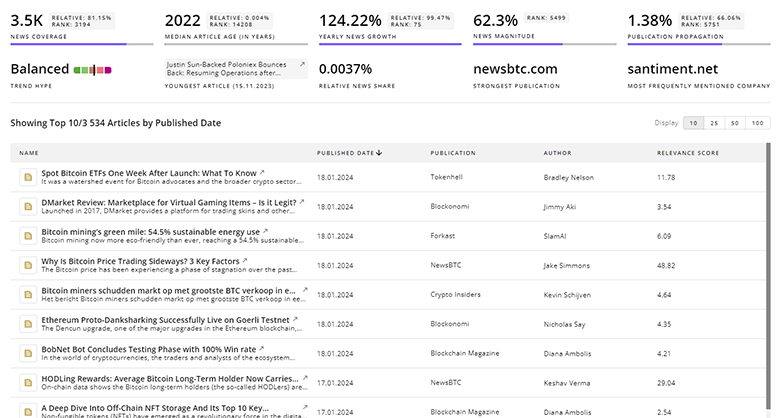
1.7K Data Annotation News Articles
Discover Latest Data Annotation Articles, News Magnitude, Publication Propagation, Yearly Growth, and Strongest Publications
Executive Summary
The data annotation sector is experiencing robust growth, driven by the surge in AI and ML model development across industries. The sector is marked by a commitment to quality, diversity, and innovation in data labeling techniques. Companies are leveraging state-of-the-art technologies and methodologies to provide accurate and efficient annotation services essential for the deployment of AI applications. As the sector continues to expand, it will play an important role in enabling AI-driven solutions that enhance business operations and decision-making processes. The future of data annotation promises further advancements in technology and an even greater impact on the accuracy of AI and data management applications.
We seek partnerships with industry experts to deliver actionable insights into trends and tech. Interested? Let us know!